Sunday, April 22, 2012
Wednesday, April 18, 2012
Data Visualization / Social Media Business Models
Google Motion Charts
Blog By Stepehen Few : http://www.perceptualedge.com/blog/
Teradata link : Microstrategy
How do we make money for the Social Media ?
Advertisement. How is Google making money and how have they monetized that ? Taking your own data and selling it to you. Ad based revenue is the primariy medium to monetize social media. Posting ads on display network
Google Ad-sense Program.
What's the point of Google+
Refinement of search results
Opportunity to get more and more data about you
Facebook model
Advertising
Facebook Vs Google+
User Data Vs Search Data
Facebook know so much more about you
When / Where / What / Music / Family / Books / Kids / Gender / Photos .... Google wanted this kind of data that is why Google+ was started
Google acquestuin of Youtube
Facebook acquisition of Instagram
Teradata link : Microstrategy
How do we make money for the Social Media ?
Advertisement. How is Google making money and how have they monetized that ? Taking your own data and selling it to you. Ad based revenue is the primariy medium to monetize social media. Posting ads on display network
Google Ad-sense Program.
What's the point of Google+
Refinement of search results
Opportunity to get more and more data about you
Facebook model
Advertising
Facebook Vs Google+
User Data Vs Search Data
Facebook know so much more about you
When / Where / What / Music / Family / Books / Kids / Gender / Photos .... Google wanted this kind of data that is why Google+ was started
Google acquestuin of Youtube
Facebook acquisition of Instagram
- Easy to upload photos
- Increased User Base
Facebook Insights
Twitter Business Model
FireHose -> Access to tweets in real time
Brand and sponcered
Fremium Business Model
Linked in targeting Companies
Huge market for recruiters. Attractive candidates for future recruitment.
Virtual Goods Model
Location Based Business Model
Four Square
Twitter + American Express + Four Square Partnership
Group on and living social
URL Shortner - BitURL
Diffusion pattern in News Media
Yelp / Trip Advisor
Selling opinions to third party !
Sopnsored Vs Regular Link
What is the Unique Value Proposition and sustain you over time
Tuesday, April 17, 2012
Social Media Twitter Project and more on Dimensional Modelling
Week end Before April 9th is the day to run through the presentation with Feedback for the GOMC !!!
Profile it and clean up. AYFG operational database and put in into SSIS
Build a data mart - OLAP, Visualize and Dashboard
Network Analysis - Advanced Analysis
Del - Dashboards and Network Analysis reports
Going to submit the video
May 7th is the deliverable date !
OLAP Reporting on Monday
SSIS
Dimensional Modelling
MON -> MS BI Suite OLAP Demo
WEd -> OBIE Suite Demo
Difference of working of the OBIE and MSBI tool. Create a data-mart
Next HW : Analysis of the airline Db (Tean)
Next HW : Info-graphic resume (Indi)
Next HW : Learnings of the class(Team)
Social Media Analytics
Facts for AYFG :
Quantity
Items
Time
Members
Gym
Customers
Store
Granularity : Specific Item across a particular gym at particular time. You are able to aggregate it across multiple facts.
Dimensions act as a mechanism to describe individual fact.
What happens when promotions are going on ? What if more promotions are going on simultaneously
You can have promotions as a dimensions. All products might not have dimensions
Promotion Dimension Table
PSK
Name
Details
Can be a simple price discount. Buy 1 Get 1 free !
You might not want to have a separate table.
Factless Fact Table
ISK -> Item
SSK - Store
TSK -> Time
PSK -> Promotion
Set difference will tell you what was on sale and did not sell or the other way.
A factless fact table is a table that contains nothing but dimensional keys.
There are two types of factless tables. One is for capturing the event. An event establishes the relationship among the dimension members from various dimension but there is no measured value. The existence of the relationship itself is the fact.
This type of fact table itself can be used to generate the useful reports. You can count the number of occurrences with various criteria. For example, you can have a factless fact table to capture the student attendance (the example used by Ralph). The following questions can be answered:
All the queries are based on the COUNT() with the GROUP BY queries. I think that the interesting metrics are the nested GROUP BY so you can first count and then apply other aggregate functions such as AVERAGE, MAX, MIX.
The other type of factless table is called Coverage table by Ralph. It is used to support negative analysis report. For example a Store that did not sell a product for a given period. To produce such report, you need to have a fact table to capture all the possible combinations. You can then figure out what is missing.
No tuple appears if there is no sale/purchase. You can have NULL values for surrogate keys.
Profile it and clean up. AYFG operational database and put in into SSIS
Build a data mart - OLAP, Visualize and Dashboard
Network Analysis - Advanced Analysis
Del - Dashboards and Network Analysis reports
Going to submit the video
May 7th is the deliverable date !
OLAP Reporting on Monday
SSIS
Dimensional Modelling
MON -> MS BI Suite OLAP Demo
WEd -> OBIE Suite Demo
Difference of working of the OBIE and MSBI tool. Create a data-mart
Next HW : Analysis of the airline Db (Tean)
Next HW : Info-graphic resume (Indi)
Next HW : Learnings of the class(Team)
Social Media Analytics
Facts for AYFG :
Quantity
Items
Time
Members
Gym
Customers
Store
Granularity : Specific Item across a particular gym at particular time. You are able to aggregate it across multiple facts.
Dimensions act as a mechanism to describe individual fact.
What happens when promotions are going on ? What if more promotions are going on simultaneously
You can have promotions as a dimensions. All products might not have dimensions
Promotion Dimension Table
PSK
Name
Details
Can be a simple price discount. Buy 1 Get 1 free !
You might not want to have a separate table.
Factless Fact Table
ISK -> Item
SSK - Store
TSK -> Time
PSK -> Promotion
Set difference will tell you what was on sale and did not sell or the other way.
A factless fact table is a table that contains nothing but dimensional keys.
There are two types of factless tables. One is for capturing the event. An event establishes the relationship among the dimension members from various dimension but there is no measured value. The existence of the relationship itself is the fact.
This type of fact table itself can be used to generate the useful reports. You can count the number of occurrences with various criteria. For example, you can have a factless fact table to capture the student attendance (the example used by Ralph). The following questions can be answered:
- Which class has the least attendance?
- Which teachers taugh the most students?
- What is the average number of attendance of a given course?
All the queries are based on the COUNT() with the GROUP BY queries. I think that the interesting metrics are the nested GROUP BY so you can first count and then apply other aggregate functions such as AVERAGE, MAX, MIX.
The other type of factless table is called Coverage table by Ralph. It is used to support negative analysis report. For example a Store that did not sell a product for a given period. To produce such report, you need to have a fact table to capture all the possible combinations. You can then figure out what is missing.
No tuple appears if there is no sale/purchase. You can have NULL values for surrogate keys.
Social media can have Fact-less Fact Table. Need to think about it !
Degenerated Key
Market Basket Analysis and associated promotions
Market Basket Analysis is a modelling technique based upon the theory that if you buy a certain group of items, you are more (or less) likely to buy another group of items. For example, if you are in an English pub and you buy a pint of beer and don't buy a bar meal, you are more likely to buy crisps (US. chips) at the same time than somebody who didn't buy beer.
FACTS -> As granular as possible
Dimensions
AYFD Datamarts
Eller GOMC Presentation
GOMC
20 minutes slot(10 mins of presenatations)
Deadlines will be posted. PPT submission 2 days before presentation. June 15th is the last day to submit.
Generally within 2 weeks after completion of your campeign.
Video of the presentation screened / Points of Presentation (Check List)
What stood out of this presentation ?
Divided up the presentation, Under graduate team. Passionate about the cause !
Analytically thinking. Show numbers on every slide to justify numbers.
Scenario before and after.
Clear Conversion Goals !
Why only a particular number of volunteers ? .. What are u going to do with excess volunteers !
Numbers justifying numbers relating to business goals.
Practice and split up presentations ! Dr.Ram is ready to meet up individually with the PPT. She will help us to review the report
ROI calculation is very important. Needs to be put
Try to quantify the ROI.
Time your self.
Introduce your client and thank them
20 minutes slot(10 mins of presenatations)
Deadlines will be posted. PPT submission 2 days before presentation. June 15th is the last day to submit.
Generally within 2 weeks after completion of your campeign.
Video of the presentation screened / Points of Presentation (Check List)
- Choosing the Client
- Why NGO
- Why OPCS
- OPCS's Exisiting Website
- Campeign Structure
- NAme Campeigns connect to website, Adwords / population connections
- Goals / Volunteering .. Connect to Mission Statement GAOLS .. 20% more PAge views / Convesions
- Revenue generation . 20% to download wish list
- Matriz used .. high traffic
- Campeign Strategy .. What did u do / Geo Targeting
- Budget Allocation
- Weekly objectives / Budget allocation
- First week Disappointment / Ad rank -> Placement -> Conversion
- Required drastic conversion
- Increased Max CPC
- Problems and Resolutions
- First major changes -> Change in Landing pages / Conversion
- Second Major Changes -> Ad group Changes / Changes to download the calendar etc
- Redefine conversion
- Third Major change -> More descriptive change in the ad using key words
- Increased daily budget Adding new keywords
- Week Wise events .. Focus on primary goals
- Overall Results
- Impressions VS CTR
- Why we chose a particular schedule for the campaigns
- Impact of a particular event ?
- When were most conversion
- How much money is earned per conversions ?
- Wish List ?
- Campaign and ad-words wise objectives !
- Increase in page traffic
- Future Recommendations Budget / Social Platform / Receptive audience / Direct to all audience / Optimize Marketing strategy
- Learning outcomes
- Client Relationships
- Blog snapshot
Questions :
- Comparison with previous three weeks ?
- Biggest challenge ?
- What did u do when u cannot make change to website ?
- What matters is what did u do to deal with the challange
What they did good :
- Clearly defines conversion goals ?
- What conversion meant ?
- Numbers ? Forecast ?
So many downloads, Page views, Volunteers
What stood out of this presentation ?
Divided up the presentation, Under graduate team. Passionate about the cause !
Analytically thinking. Show numbers on every slide to justify numbers.
Scenario before and after.
Clear Conversion Goals !
Why only a particular number of volunteers ? .. What are u going to do with excess volunteers !
Numbers justifying numbers relating to business goals.
Practice and split up presentations ! Dr.Ram is ready to meet up individually with the PPT. She will help us to review the report
ROI calculation is very important. Needs to be put
Try to quantify the ROI.
Time your self.
Introduce your client and thank them
Staging area data warehouse basics
Staging areas are used to temporarily store data extracted from source systems so that it can be prepared for subsequent cleansing and transformation.
Table structures usually are very similar to the table structure or file layouts in the source systems and are a series of “flat” table structures. In other words, they have no indexes and no relationships to other tables in the staging area.
Data structures may be designed to include:
- In-scope data e.g. only data in scope is loaded in the staging area; or
- All data, e.g. all data in the source table or files is loaded in the staging area and subsequent transformations eliminate non-essential data.
- Dates might be transformed from mm/dd/yy to yy/mm/dd; or
- Numeric values might be converted to varchar (if not needed for reporting).
Why Union Vs Join ?
Consider all related dimensions while loading it
Need to IDentify te Cube that u aregoing to build
How logical is your DB ?
Slowly Changing Dimensions
OLAP Vs OLTP
Slowly Changing Dimensions
Type 0 -> No Changes Ever, Ostrich technique
Type 1 -> U go and update the attribute. Change the brand. Completely lost the history. Very Simple technique. But difficult to manage history !. If you are not interested in history
Type 2 -> Surrogate Key should be Unique. Create a NEW surrogate Key and keep this history data. Every time attribute value changes, add a new tuple in the dimension table with NEW surrogate key. Old facts under old surrogate key. New facts under NEW surrogate key. Unlimited number of changes under new territory.
Type 3 -> Add a New Column
Junk Dimension
In a data warehouse, a dimension is a data element that categorizes each item in a data set into non-overlapping regions. A data warehouse dimension provides the means to "slice and dice" data in a data warehouse. Dimensions provide structured labeling information to otherwise unordered numeric measures. For example, "Customer", "Date", and "Product" are all dimensions that could be applied meaningfully to a sales receipt. A dimensional data element is similar to a categorical variable in statistics.
Types of Facts
There are three types of facts:
Additive: Additive facts are facts that can be summed up through all of the dimensions in the fact table.
Semi-Additive: Semi-additive facts are facts that can be summed up for some of the dimensions in the fact table, but not the others.
Non-Additive: Non-additive facts are facts that cannot be summed up for any of the dimensions present in the fact table.
Slowly Changing Dimensions
Type 0 -> No Changes Ever, Ostrich technique
Type 1 -> U go and update the attribute. Change the brand. Completely lost the history. Very Simple technique. But difficult to manage history !. If you are not interested in history
Type 2 -> Surrogate Key should be Unique. Create a NEW surrogate Key and keep this history data. Every time attribute value changes, add a new tuple in the dimension table with NEW surrogate key. Old facts under old surrogate key. New facts under NEW surrogate key. Unlimited number of changes under new territory.
Type 3 -> Add a New Column
Junk Dimension
In a data warehouse, a dimension is a data element that categorizes each item in a data set into non-overlapping regions. A data warehouse dimension provides the means to "slice and dice" data in a data warehouse. Dimensions provide structured labeling information to otherwise unordered numeric measures. For example, "Customer", "Date", and "Product" are all dimensions that could be applied meaningfully to a sales receipt. A dimensional data element is similar to a categorical variable in statistics.
A star schema that has many dimensions is sometimes called a centipede schema[2]. Having dimensions of only a few attributes, while simpler to maintain, results in queries with many table joins and makes the star schema less easy to use.
There are three types of facts:
Additive: Additive facts are facts that can be summed up through all of the dimensions in the fact table.
Semi-Additive: Semi-additive facts are facts that can be summed up for some of the dimensions in the fact table, but not the others.
Non-Additive: Non-additive facts are facts that cannot be summed up for any of the dimensions present in the fact table.
Monday, April 16, 2012
Visualization
Learnings :
Dangerous to use Average Data.
OLAP reporting we aggregate. Aggregate can give u a different picture. Drill down capability is very critical.
Different types of graphics - Different visual representation.
Explain behavior - Seeing certain patterns. Why the numbers the way they are
Volume of data
Accessibility of data
Cleaning the data is very critical
Ability to drill down to extreme level
Infographics
Wednesday, April 11, 2012
Balanced Scorecard / Strategy Maps / Usage
The Balanced Scorecard (BSC) is a strategic performance management tool - a semi-standard structured report, supported by proven design methods and automation tools, that can be used by managers to keep track of the execution of activities by the staff within their control and to monitor the consequences arising from these actions. Kaplan and Norton cam up with this idea.
Evaluation not just based on Financial performance you might not be able to sustain performance .
Apart from Fin perspective there are other perspectives which can be seen as follows:
Profit = RASM - CASM ... Maximize the difference
Evaluation not just based on Financial performance you might not be able to sustain performance .
Apart from Fin perspective there are other perspectives which can be seen as follows:
- Customer
- Learning and Growth
- Internal Process -> Investing in our learning and growth. Positioning and Training etc
Can be applied at any level of the company !
Example of Zappos. Managing and maintaining preformance. How do we do it ? How do we apply ?
Strategy Maps - How are you going to achieve this ? What are the series of steps and actions to achieve the level of performance.
Airline Industry Models :
Hub and Spoke Model - American, Delta, United, Continental
Point to Point Model - Southwest
Airline Industry Pressure Points :
- Competition
- Fuel prices
- Federal regulation
- Security
Theme for the strategy Maps for Southwest : Low Cost Efficiency !
2 Matrix in Airline Industry
CASM
RASM
CASM is a commonly used measure of unit cost in the airline industry. CASM is expressed in cents to operate each seat mile offered, and is determined by dividing operating costs by ASMs. This number is frequently used to allow a cost comparison between different airlines or for the same airline across different time periods (say for one year vs the preceding year). A lower CASM means that it is easier for the airline to make a profit, as they have to charge less to break even.
Monday, March 26, 2012
STAR Schema(AYEG Case) and Microsoft BI
OLAP VS OLTP
OLPT and OLAP are complementing technologies. You can't live without OLTP: it runs your business day by day. So, using getting strategic information from OLTP is usually first “quick and dirty” approach, but can become limiting later.
This post explores key differences between two technologies.
OLTP stands for On Line Transaction Processing and is a data modeling approach typically used to facilitate and manage usual business applications. Most of applications you see and use are OLTP based.
OLAP stands for On Line Analytic Processing and is an approach to answer multi-dimensional queries. OLAP was conceived for Management Information Systems and Decision Support Systems but is still widely underused: every day I see too much people making out business intelligence from OLTP data!
Starschemas are not normalized. Not updating and deleting tuples that u do in normal database. Facitilates queries instead of other operation. When selecting there is not update to index which makes use of Index easier.
GRANULARITY - data warehouse typically stores data in different levels of granularity or summarization, depending on the data requirements of the business. If an enterprise needs data to assist strategic planning, then only highly summarized data is required. The lower the level of granularity of data required by the enterprise, the higher the number of resources (specifically data storage) required to build the data warehouse. The different levels of summarization in order of increasing granularity are:
- Current operational data
- Historical operational data
- Aggregated data
- Metadata
AYEG
Revenue Sources:
Pro Shop and juice bar
Membership and Day Passes
Problem : How to generate more revenue.Which set of customers should the gym target. Has the customer pattern changed over time ?
BI is all about how to maximize revenue based on internal or extranal data
Now Organize corp events. Customer pay fixed fees
What are the thoughts about
Need to have a look at the OLTP data. Need to look at the trends and patterns. Good point to start with. Need to relate with DW Tool Kit by Ralph and Kimball. Can the abve questions be answered using OLTP DB. Yes ... Need to do some messaging the data over time.
For some questions you need the historical data. Even if u have multiple sources of data you need to dump it into single datawarehouse.
A data mart is the access layer of the data warehouse environment that is used to get data out to the users. The data mart is a subset of the data warehouse which is usually oriented to a specific business line or team.
This can also be done by Virtual Integration. This could be a step towards complete integration.
With a virtual data warehouse, you don't have to go through all the hard work of integrating data, rummaging around old files and trying to understand what some programmer back in the 1960s had in mind. You don't need to take up space with replicated data. What a lovely idea. You just string some files together and access them as if they were a data warehouse.
Apache Cassandra is an open source distributed database management system used by Hadoop.
A dimension is a structure that categorizes data in order to enable users to answer business questions. Commonly used dimensions are customers, products, and time. For example, each sales channel of a clothing retailer might gather and store data regarding sales and reclamations of their Cloth assortment. The retail chain management can build a data warehouse to analyze the sales of its products across all stores over time and help answer questions such as:
What is the effect of promoting one product on the sale of a related product that is not promoted?
What are the sales of a product before and after a promotion?
How does a promotion affect the various distribution channels?
The data in the retailer's data warehouse system has two important components: dimensions and facts. The dimensions are products, customers, promotions, channels, and time. One approach for identifying your dimensions is to review your reference tables, such as a product table that contains everything about a product, or a promotion table containing all information about promotions. The facts are sales (units sold) and profits. A data warehouse contains facts about the sales of each product at on a daily basis.
A typical relational implementation for such a data warehouse is a Star Schema. The fact information is stored in the so-called fact table, whereas the dimensional information is stored in the so-called dimension tables. In our example, each sales transaction record is uniquely defined as for each customer, for each product, for each sales channel, for each promotion, and for each day (time).
BI Rules Learnt in today's Class :
- Facts are quantitative attributes that can be added across every dimension !!!
- Items are not quantitative. Quantity and $ amount are quantitative.
- Dimension Tables are short and Fat
- Fact table are tall(exhaustive) and skinny(very few attributes)
- Surrogate key represent dimensions.
Assumption : Every GYM has a local database. Think beyond something which is not there in a regular database.
Dimensions :
Time : Day, Hour
Space : Location where the sale is happening. Location of the Gym. Gym Dimension
Membership : Type
Items : Things that you buy, Products
Facts:
Sales : $ sales amountTotal # of member :
Quantity has Members, Time and Item dimensions.
What do you do about memberships ? Separate Schema for memberships. Only include retail sales.
Attributes for membership : Types of membership, Membership ID
Importance of Surrpogate
Data warehouses typically use a surrogate, (also known as artificial or identity key), key for the dimension tables primary keys. They can use Infa sequence generator, or Oracle sequence, or SQL Server Identity values for the surrogate key. It is useful because the natural primary key (i.e. Customer Number in Customer table) can change and this makes updates more difficult.
Slice and Dice
The ability to move between different combinations of dimensions when viewing data with an OLAP browser.
Staging -> Operational Data Store -> Datawarehouse
Wednesday, March 21, 2012
Dimensional Modelling
Considers a General Departmental Store. This is in third normal form. Dimensional modeling (DM) is the name of a set of techniques and concepts used in data warehouse design. It is considered to be different from entity-relationship modeling (ER). Dimensional Modeling does not necessarily involve a relational database. The same modeling approach, at the logical level, can be used for any physical form, such as multidimensional database or even flat files. According to data warehousing consultant Ralph Kimball, DM is a design technique for databases intended to support end-user queries in a data warehouse. It is oriented around understandability and performance. According to him, although transaction-oriented ER is very useful for the transaction capture, it should be avoided for end-user delivery.
CUSTOMERS (CID, CName, Address, Phone,...,...)
PRODUCT (PId, PName, Brand, Catagory, Size ,...,...)
ORDERS(OId, Date, Total, Cash/Credit,...,...)
ORDERID(OId, ProdID, ProductQty, Line#, Amount)
This is OLTP Database. Inserting, Deleting, Updating and querying data for reporting. Now you need to do more analysis. Need to find seasonality, the demand, patters.
You can do that using the OLTPdatabase, but it will be a big problem because of joins / aggregate queries. Ways to do aggregate query :
Group By Cust ID, Product_ID
Sum (Quantity)
then slice and dice it. But its hard to do it because of the nature if data.
Indexing speed up retrieval but overheads are costtly. When you have too many indexes(Dense / Sparse Index) ... The system might slow down .. Insert / Delete queries might slow down. Every time there is a transcriptional update the Index need to update.
Benefits of the dimensional modeling are following:
Understandability - Compared to the normalized model, the dimensional model is easier to understand and more intuitive. In dimensional models, information is grouped into coherent business categories or dimensions, making it easier to read and interpret. Simplicity also allows software to navigate databases efficiently. In normalized models, data is divided into many discrete entities and even a simple business process might result in dozens of tables joined together in a complex way.
Query performance - Dimensional models are more denormalized and optimized for data querying, while normalized models seek to eliminate data redundancies and are optimized for transaction loading and updating. The predictable framework of a dimensional model allows the database to make strong assumptions about the data that aid in performance. Each dimension is an equivalent entry point into the fact table, and this symmetrical structure allows effective handling of complex queries. Query optimization for star join databases is simple, predictable, and controllable.
Extensibility - Dimensional models are extensible and easily accommodate unexpected new data. Existing tables can be changed in place either by simply adding new data rows into the table or executing SQL alter table commands. No queries or other applications that sit on top of the Warehouse need to be reprogrammed to accommodate changes. Old queries and applications continue to run without yielding different results. But in normalized models each modification should be considered carefully, because of the complex dependencies between database tables.
In Star Schema you have un normalized tables.
FACTS are additive and can add across all it's dimendions
DIMENSIONS
What kinds of questions you are going to ask. Sales in terms of customers / geography / products. Central to sales is the $ amount. What is the $ amount for the order. Fact as the quantitative attribute. Most Data Whorehouse has quantitative attributes.
Customer , Product become dimensions. One to man relations between dimensions and fact. Finer granularity of the fact more the detailed analysis I can do.
Granularity is usually mentioned in the context of dimensional data structures (i.e., facts and dimensions) and refers to the level of detail in a given fact table. The more detail there is in the fact table, the higher its granularity and vice versa. Another way to look at it is that the higher the granularity of a fact table, the more rows it will have.
Dimension is a term in data management and data warehousing that refers to logical groupings of data such as geographical location, customer information, or product information. Slowly Changing Dimensions (SCDs) are dimensions that have data that changes slowly, rather than changing on a time-based, regular schedule.
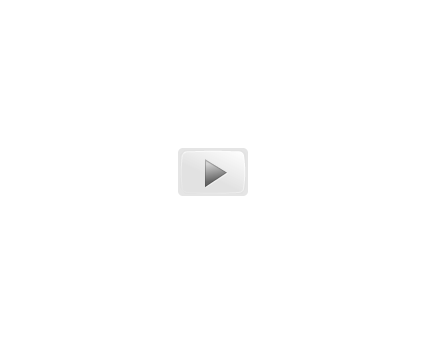
Facts are analyzed based in Dimensions.
Monday, March 19, 2012
Data Quality , Profiling , Clensing, MDM and Data Forensics
Accurate data does not come for free. It requires careful attention to the design of systems, constant monitoring of data collection, and aggressive actions to correct problems that generate or propagate inaccurate data.
-Jack Olsen Data Quality: The Accuracy Dimension
Data Warehouse (The Data Warehouse) is a database of unique data structure that allows relatively quick and easy performance of complex queries over large amounts of data.
Data put into warehouse must be clean. Companies spend 80% of effort in cleaning and profiling. Most companies have problem with the quality of Data.
Data Profiling -> Uncovering the Business Rules from Data Given.
Problems with Profiling:
Lack of
Approach
Study the Schema properly and then decide the test strategy and answer the following questions :
- How many distinct values are present
- Primary key capability of a field
- Format of a particular field
- Business Rules - 1-> Male 2-> Female OR M-> Male F-> Female
- Checks on various fields
Checks on individual data fields :
Tests :
(1) Primary Key and Foreign Key Tests
- Missing primary keys / Primary Key Violation
- Unique ness across the table
- Email Address should have a NON NULLValue
(2)Combinations Of Attributes
- Referential Integrity
- Relates to Criminality
- Employee works in just one Dept. Employee had just one Dept ID assigned to him. Dept can have zero to maximum employees. So the combination of attribute check will point this out. There should be no missing values for a table.
- Dept can have No employees. Need to go back to documentation and check the criminality of the tables
- Business rule defined on Salary depending on role . Minimum Salary depends on level
- Salary field withing minimum and Maximum ranges
- Restriction related to dept number. Need to have a framework in mind. Individual attributes and different attributes across tables. Problem is most of the companies do not have documentation. You need to have a schema and then reverse engineer. This makes profiling complex.
- Business rules are embedded in the procedures and look at what procedures are telling you.
- Individual Attribute Tests
- Aggregate Columns
Reverse Engineer ER Model and check for businesss rules that apply.
Checks all realted to SQL.. Difference between Unique and Distinct
Reference to Ataccama DQ tool.
Write own programs to do tests. Data profiling tool to
Business rules that govern individual attribute
Range Check on Salary field
Why Errors Creep in ?
- Data comes from multiple sources and multiple sources might not have the constraints implemented
- Pause constraints function in Oracle and bring in data from other tables
- When u start you have high quality data but as time passes the quality deteriorates.
Where can u find the Business rules of a company ?
- Most relational DB has catalog which has constraints.
- System catalog has tremendous amount of business information
- Application Layer programs. Many companies embed business rules in application layer programs
- E.g Employee can lead only one project. It can be used ref integrity.
- User can also be very critical who can be capable to give info about business rules
This is called Data Forensics :
- If company insists on investigating every aspect of business intelligence reporting errors then we are talking about data forensic. It is mandatory to be aware about reporting mistakes that happened but too big focus on every detail of generated error (who made mistake, who signed, who defined process, was everything under procedure, what is the error impact and etc.)
Data profiling is the process of examining the data available in an existing data source (e.g. a database or a file) and collecting statistics and information about that data. The purpose of these statistics may be to:
- Find out whether existing data can easily be used for other purposes
- Improve the ability to search the data by tagging it with keywords, descriptions, or assigning it to a category
- Give metrics on data quality, including whether the data conforms to particular standards or patterns
- Assess the risk involved in integrating data for new applications, including the challenges of joins
- Assess whether metadata accurately describes the actual values in the source database
- Understanding data challenges early in any data intensive project, so that late project surprises are avoided. Finding data problems late in the project can lead to delays and cost overruns.
- Have an enterprise view of all data, for uses such as master data management where key data is needed, or data governance for improving data quality.
What is Master Data Management ? (Interview Question)
- Its not a tool but a process as a whole. Its a costly effort. It takes a while and cannot see the result(Dash Board) very soon. That takes time. MDM happens behind the scene.
- In computing, master data management (MDM) comprises a set of processes and tools that consistently defines and manages the master data-of an organization (which may include reference data). MDM has the objective of providing processes for collecting, aggregating, matching, consolidating, quality-assuring, persisting and distributing such data throughout an organization to ensure consistency and control in the ongoing maintenance and application use of this information.
Data Quality
- How well Data matches business rules
- Integrity / Reliability
Aspects of data quality include:
- Accuracy
- Completeness
- Update status
- Relevance
- Consistency across data sources
- Reliability
- Appropriate presentation
- Accessibility
Most Companies make money by offering services and not in licensing. Ask for different stages in MDM. Services which are bring provided on MDM. Training employees on MDM process ? Road map / Tools / Functionalities on MDM.
Friday, February 17, 2012
Intro to BI :(Lecture 1)
Business intelligence (BI) mainly refers to computer-based techniques used in identifying, extracting,and analyzing business data, such as sales revenue by products and/or departments, or by associated costs and incomes.
BI technologies provide historical, current and predictive views of business operations. Common functions of business intelligence technologies are reporting, online analytical processing, analytics, data mining, process mining, complex event processing, business performance management, benchmarking, text mining and predictive analytics.
As per our BI instructor Prof.Dr Ram, Business intelligence aims to support better business decision-making. Thus a BI system can be called a decision support system. Though the term business intelligence is sometimes used as a synonym for competitive intelligence, because they both support decision making, BI uses technologies, processes, and applications to analyze mostly internal, structured data and business processes while competitive intelligence gathers, analyzes and disseminates information with a topical focus on company competitors. Business intelligence understood broadly can include the subset of competitive intelligence.
Hope to learn lots of new concepts and exciting funda's of BI.
Subscribe to:
Posts (Atom)