Considers a General Departmental Store. This is in third normal form. Dimensional modeling (DM) is the name of a set of techniques and concepts used in data warehouse design. It is considered to be different from entity-relationship modeling (ER). Dimensional Modeling does not necessarily involve a relational database. The same modeling approach, at the logical level, can be used for any physical form, such as multidimensional database or even flat files. According to data warehousing consultant Ralph Kimball, DM is a design technique for databases intended to support end-user queries in a data warehouse. It is oriented around understandability and performance. According to him, although transaction-oriented ER is very useful for the transaction capture, it should be avoided for end-user delivery.
CUSTOMERS (CID, CName, Address, Phone,...,...)
PRODUCT (PId, PName, Brand, Catagory, Size ,...,...)
ORDERS(OId, Date, Total, Cash/Credit,...,...)
ORDERID(OId, ProdID, ProductQty, Line#, Amount)
This is OLTP Database. Inserting, Deleting, Updating and querying data for reporting. Now you need to do more analysis. Need to find seasonality, the demand, patters.
You can do that using the OLTPdatabase, but it will be a big problem because of joins / aggregate queries. Ways to do aggregate query :
Group By Cust ID, Product_ID
Sum (Quantity)
then slice and dice it. But its hard to do it because of the nature if data.
Indexing speed up retrieval but overheads are costtly. When you have too many indexes(Dense / Sparse Index) ... The system might slow down .. Insert / Delete queries might slow down. Every time there is a transcriptional update the Index need to update.
Benefits of the dimensional modeling are following:
Understandability - Compared to the normalized model, the dimensional model is easier to understand and more intuitive. In dimensional models, information is grouped into coherent business categories or dimensions, making it easier to read and interpret. Simplicity also allows software to navigate databases efficiently. In normalized models, data is divided into many discrete entities and even a simple business process might result in dozens of tables joined together in a complex way.
Query performance - Dimensional models are more denormalized and optimized for data querying, while normalized models seek to eliminate data redundancies and are optimized for transaction loading and updating. The predictable framework of a dimensional model allows the database to make strong assumptions about the data that aid in performance. Each dimension is an equivalent entry point into the fact table, and this symmetrical structure allows effective handling of complex queries. Query optimization for star join databases is simple, predictable, and controllable.
Extensibility - Dimensional models are extensible and easily accommodate unexpected new data. Existing tables can be changed in place either by simply adding new data rows into the table or executing SQL alter table commands. No queries or other applications that sit on top of the Warehouse need to be reprogrammed to accommodate changes. Old queries and applications continue to run without yielding different results. But in normalized models each modification should be considered carefully, because of the complex dependencies between database tables.
In Star Schema you have un normalized tables.
FACTS are additive and can add across all it's dimendions
DIMENSIONS
What kinds of questions you are going to ask. Sales in terms of customers / geography / products. Central to sales is the $ amount. What is the $ amount for the order. Fact as the quantitative attribute. Most Data Whorehouse has quantitative attributes.
Customer , Product become dimensions. One to man relations between dimensions and fact. Finer granularity of the fact more the detailed analysis I can do.
Granularity is usually mentioned in the context of dimensional data structures (i.e., facts and dimensions) and refers to the level of detail in a given fact table. The more detail there is in the fact table, the higher its granularity and vice versa. Another way to look at it is that the higher the granularity of a fact table, the more rows it will have.
Dimension is a term in data management and data warehousing that refers to logical groupings of data such as geographical location, customer information, or product information. Slowly Changing Dimensions (SCDs) are dimensions that have data that changes slowly, rather than changing on a time-based, regular schedule.
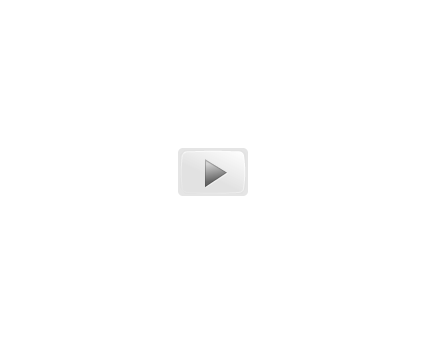
Facts are analyzed based in Dimensions.
No comments:
Post a Comment